Small, not big data key to working out what consumers want
- Written by Peter Swan Professor of Finance at UNSW Australia Business School
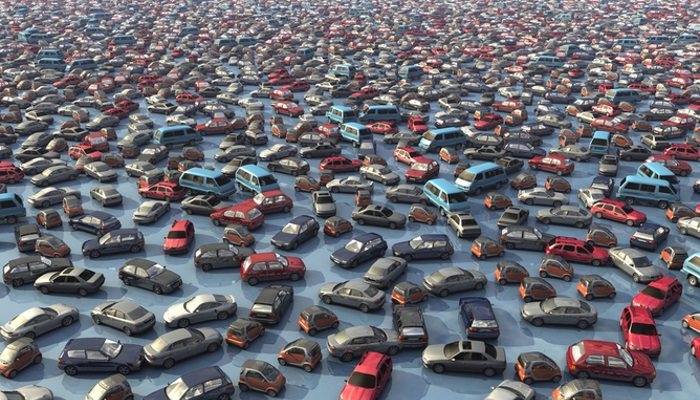
Corporations everywhere are hoovering up petabytes of data in a bid to understand and predict consumer preferences. But what if they’re missing the point, and should instead focus on “small data”?
First, some history. In 1844, French engineer Jules Dupuit developed a concept that later became known as consumer surplus. He posed what has become a familiar problem even today: if, for example, the government is planning a new Sydney Harbour crossing, should it be built and, if so, how should the cost be recovered from users?
Dupuit proposed that if the maximum amount that users were willing to pay for a bridge exceeded the necessary cost outlay then society would gain. Costs would be recovered by a system of discriminatory charges on different classes of users reflecting their willingness to pay.
Many, if not all, public goods provided by governments that we take for granted owe their justification, if not their existence, to the powerful concept of consumer surplus. This includes Australia’s first national park, now the Royal National Park, established in 1879.
Strange as it may seem today, 19th century Sydneysiders placed “enjoyment of the outdoors” ahead of conservation when the park was established.
But parks cost the state money and then, as today, there was no entry charge. Why then did our forebears place a value on something that was then unmeasurable, “enjoying the outdoors”, which they thought clearly exceeded the cost of establishing the park?
Some 70 years later the answer could be found in consumer surplus.
Measuring the unmeasurable
In the 1940s the US National Park Service was looking for a rationale to justify its existence. It did so by attempting to measure the value placed by park users on recreational benefits, something that was not directly measurable.
In fact, the average Joe treated these attempts with disdain: measuring the unmeasurable is no more than a figment of the self-serving bureaucrat’s imagination!
The gifted economist Harold Hotelling provided a mechanism for satisfying the average Joe based on travel costs. Those who travel a long way to the park and in doing so incur large costs must have a high willingness to pay over and above any direct entry fee.
Hotelling’s insight lead to the development of “hedonic” statistical methods in which the “shadow prices” of attributes that were otherwise unmeasurable could be inferred from actual outlays.
For example, otherwise identical houses located near or far from a transport hub or a polluting factory would sell for different prices, enabling the locational advantage or disadvantage to be priced.
At about the same time, in 1948 to be precise, the future Nobel laureate Paul Samuelson invented “revealed preference” in which in principle people’s preferences can be inferred using backward induction from their choices or actions.
For example, if I purchase a combination of two apples and one banana, but I could have purchased one banana and two apples, then the former bundle is preferred to the latter.
These insights could have ushered in an era where consumer preferences were soundly extracted based on attributes from all of our actions. Cost-benefit analyses helping every aspect of our daily lives could have become the norm.
They did not. Something was missing.
Narrowing choice
Jumping forward another 50 years, online retailer Amazon invented a book recommendation tool: “those who purchased this book also purchased that”. Researchers have credited this ability of online retailers to both stock and recommend obscure books with adding a billion dollars to consumer surplus.
While seemingly very successful, these “big data” methodologies rely on past purchase history. They suffer serious drawbacks. Past purchase data may not be available; may be too expensive; may be too intrusive. Other people’s choices may not be relevant to me.
Moreover, while providing vague signals relating to the preferences of a current user, they do not capture the dollar compensation I would need to be indifferent between buying (say) the Mercedes C Class against the equivalent BMW model.
The missing ingredient is a means to identify the nature of my demands for all the attributes relevant for my individual choice of an item, and to do so in real time.
The identification of these normally invisible demands enables the creation of optimal weights that reflect my personal consumer surplus expressed in terms of attributes.
Prior to the invention of the web, it would have been impossible for people to express their preferences in real time simply by touching an item on a screen.
It is now possible to do this. For example, when buying a car consumers can compute their own consumer surplus based on performance, safety, economy, and luxury instantaneously in real time. They can price and value all of these attributes, even though individual attributes are not for sale. In real life, these attributes are bundled into what is known as a car.